The digital era has ushered in massive amounts of data and automation into the R&D landscape, fundamentally transforming how scientific research is conducted. The recent emergence of generative AI and large language models has further intensified this transformative trajectory.
Contemporary research demands the navigation of multi-dimensional datasets, the execution of intricate simulations, and the interpretation of complex experimental results. R&D data has evolved beyond mere management to become the cornerstone of a company’s innovation and competitive edge. Despite its immense potential, a significant portion of scientific data remains largely untapped. The challenges lie within the nature of the data itself.
One of the most profound outcomes of modern R&D, and concurrently one of its most challenging and thrilling aspects, is the unprecedented volume of research data available to scientists, including historical data, experiment-generated data, insights derived from predictive analysis, and more. The conundrum of aggregating and harnessing this diverse data trove is intricate and overwhelming, necessitating technological solutions.
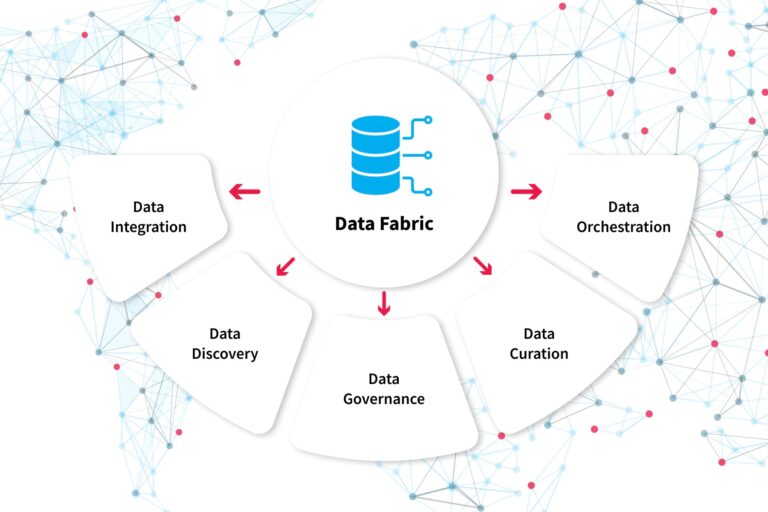
The Unstructured, Siloed Data
Many laboratories adhere to the current state of data systems, facing challenges with vast, inaccessible data stored in diverse locations and formats, hindering robust analysis and collaboration. Bottlenecks in workflows impede discovery due to scattered data across warehouses, lakes, laptops, instruments, and public databases, varying in forms like images, graphs, spectra, and genetic sequences. The continuous influx of new data exacerbates these issues.
Organizations adopting R&D data management software find their tools insufficient amid the increasing volume, velocity, and variety of research data. Many existing platforms struggle to efficiently handle today’s multifaceted scientific data requirements. The absence of an agile and flexible data system exacerbates the data silo problem, causing R&D entities to lag behind, missing out on opportunities presented by advanced technologies like machine learning and AI. This disparity directly impacts market success and share.
The solution is data fabric. A data fabric is a sophisticated design that incorporates diverse data sources and types across different environments—on-premises, cloud, or hybrid systems—forming a unified and interconnected architecture. Particularly valuable in scientific domains and research, the data fabric architecture eliminates data silos and analysis bottlenecks in R&D, enabling scientists to concentrate on their work and enhancing the pace of discovery and productivity.
The Elemental Layers of a Data Fabric
A data fabric comprises virtualization layers that securely facilitate data access, ingestion, and sharing across an organization. Forrester identifies six component layers within a data fabric:
– Data Management: This layer focuses on governance and security protocols to safeguard data.
– Data Ingestion: It integrates data from diverse sources and formats.
– Data Processing: Dedicated to filtering data, ensuring only relevant information is extracted.
– Data Orchestration: Undertakes tasks like data transformation, integration, and cleansing to make data usable.
– Data Discovery: Uncovers potential integration avenues between disparate data systems.
– Data Access: Manages permissions according to regulations and policies, providing access to interactive dashboards for users.
The advantages of implementing a data fabric in scientific R&D are substantial. If you share some of these common challenges and pain points, you need a data fabric as a part of your lab’s technology solution set:
– Internal data siloed in databases, data warehouses, data lakes, and clouds
– Large volumes of data and metadata generated across multiple projects and by different scientists
– Data dispersed on individual computers in Excel, text docs, and PDF files, unmanaged and unused
– Data from public sources that are incompatible with internal software, requiring manual wrangling
– Cumbersome and clunky analysis of structured and unstructured data
– Technical barriers to sharing and collaborating on data with collaborators
Scientific research data is expected to increase in complexity and volume, with generative AI and large language models continually advancing. A robust, flexible, and efficient data architecture is crucial for keeping pace. Integration of a data fabric enables R&D organizations to overcome current challenges and lays the foundation for future advancements.
Contact us for a discussion with an AI Materia specialist on addressing your R&D data challenges today.