For many conventional innovation-driven enterprises, scientific data is typically generated to address immediate research queries and subsequently archived for intellectual property protection, often overlooking the future potential of reusing the data for addressing related questions. Data is often considered a byproduct of R&D rather than a primary output, leading to the omission of crucial experimental details and contextual information.
The collected data is frequently inconsistent and lacks a well-structured format, posing challenges in parsing large volumes of historical data files stored in network drives or data lakes. Moreover, manual and complex experimental workflows involving coordination between multiple teams further contribute to slow and expensive data generation.
Consequently, many R&D labs possess relatively small datasets that are neither sufficiently clean nor complete for higher-level purposes, such as training machine learning models. Faced with this “small data” scenario, researchers and managers may hesitate to adopt data-driven approaches to new product development, unsure about the possibilities given the current state of their data.
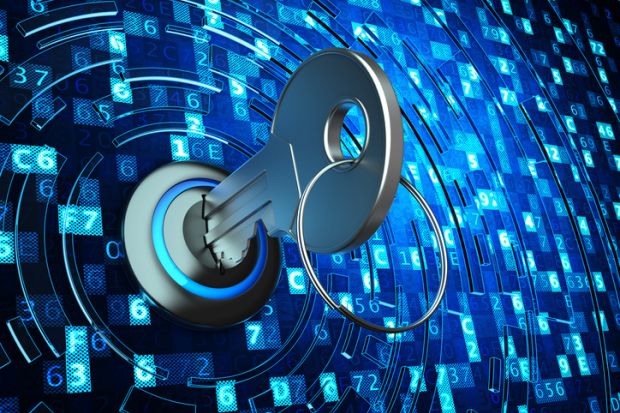
AI Materia has addressed numerous small data challenges in science-driven product development, employing diverse strategies to extract maximum value from customers’ small data for strategic innovation objectives. While there’s no one-size-fits-all solution due to the uniqueness of each R&D organization’s data and workflows, AI Materia assists in optimizing existing data and setting a path toward continuous improvement. Teams can initiate data-driven efforts with minimal data, leveraging existing domain knowledge through well-designed experimental approaches, feature engineering, informed model constraints, and improved data quality. AI Materia also evaluates current data generation workflows, prioritizing enhancements that accelerate data generation and enhance data quality using software tools to streamline labeling tasks and automate or assist users in raw data analysis.
Are you facing challenges with small-scale data in your lab?
Don’t let this hinder your adoption of data-driven approaches. In organizations where small data is prevalent, leveraging data-driven modeling and prediction can offer significant value, expediting discovery and innovation. Reach out to us today to explore solutions for your team’s small data challenges.